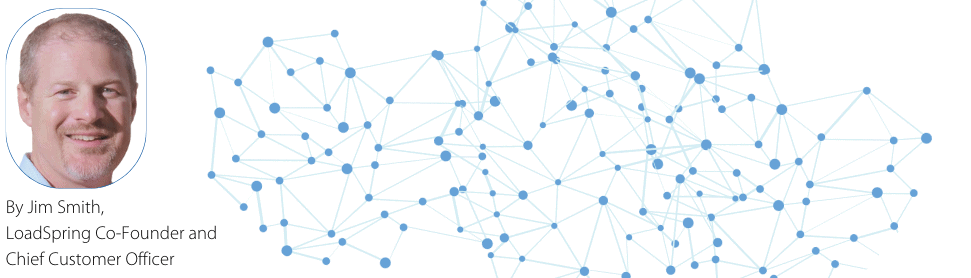
Unlocking the New Frontier: How Large Language Models and Natural Language Queries Are Revolutionizing the AEC Industry
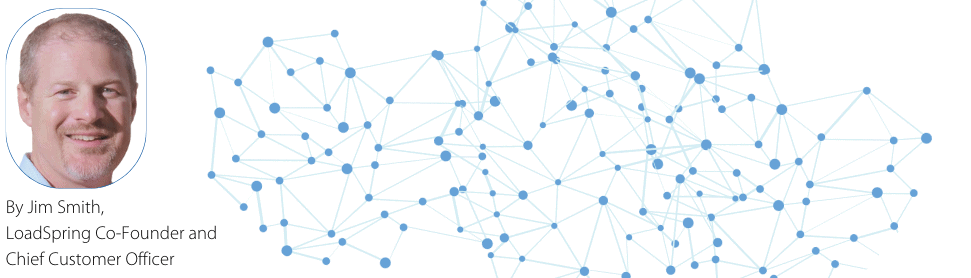
In the ever-evolving landscape of Architecture, Engineering, and Construction (AEC), the ability to effectively query and derive insights from project-intensive data is paramount. Traditionally, this process has been labor-intensive, requiring specialized technical knowledge and expertise. However, the emergence of Large Language Models (LLMs) and Natural Language Query (NLQ) has ushered in a new era, revolutionizing the way AEC companies interact with their data.
The Rise of Large Language Models
LLMs and NLQs have gained significant attention in the AEC space due to their immense capabilities in language comprehension and generation. Technologies that utilize AI to leverage LLMs, such as LoadSpring’s Talk-to-Your-Data™ (TTYD) solution, are at the forefront of transforming data querying processes for AEC companies. Furthermore, through advanced natural language processing techniques, LLMs and NLQs can now interpret and respond to human queries with remarkable precision.
Enhanced Natural Language Understanding
Traditional data querying methods often require decision makers to work with data scientists or other technical staff who are versed in specific query languages or employ strict syntax to retrieve information. However, LLMs have dramatically simplified this process by allowing users to submit queries in a more natural and human-like manner – conversational English, for example. By leveraging their understanding of context, LLMs can accurately interpret the intent behind a query even in complex and nuanced scenarios. This natural language understanding capability has made data querying accessible to a wider range of users, removing barriers to entry and empowering individuals from non-technology backgrounds to gain insights from data in real time.
Improved Query Relevance and Accuracy
LLMs have significantly raised the bar when it comes to query relevance and accuracy. These models can contextualize user queries within the dataset, considering relevant associations, relationships, and patterns. As a result, LLMs can provide more targeted and precise results, saving users time and effort in sifting through irrelevant information. By leveraging deep learning and continuous training, LLMs also have the capacity to improve their accuracy over time through exposure to more data, honing their ability to retrieve the most relevant information with each query.
It is important to note that queries conducted by LLMs are only as good and accurate as the source data. If the LLM is asked to query on inherently poor, irrelevant, dirty, or incomplete data; the results will only reflect the quality of that data.
Flexible Querying Approaches
Another transformative aspect of LLMs in data querying is their flexibility and adaptability. While traditional querying methods often rely on structured queries or predefined schemas, LLMs can handle unstructured queries with ease. Users are no longer bound by rigid query formats and can ask questions in a conversational manner, receiving responses that are coherent and informative.
Efficiency and Scalability
Technologies that utilize AI to leverage LLMs, such as LoadSpring’s Talk-to-Your-Data™ solution, can process complex queries at scale, retrieving results swiftly, consistently, and accurately, enabling rapid insights that would otherwise be time-consuming or even infeasible using traditional querying methods. LLMs and NQLs represent an exciting innovation in the field of data querying. By offering enhanced natural language understanding, improved query relevance and accuracy, flexible querying approaches, and efficient scalability, these advanced technologies have fundamentally transformed the way the AEC industry interacts with data. As LLM/NLQ technology continues to advance, we can anticipate even more significant shifts in the data querying landscape, empowering users to derive insights, make better-informed decisions, and uncover valuable patterns and relationships hidden within vast datasets.