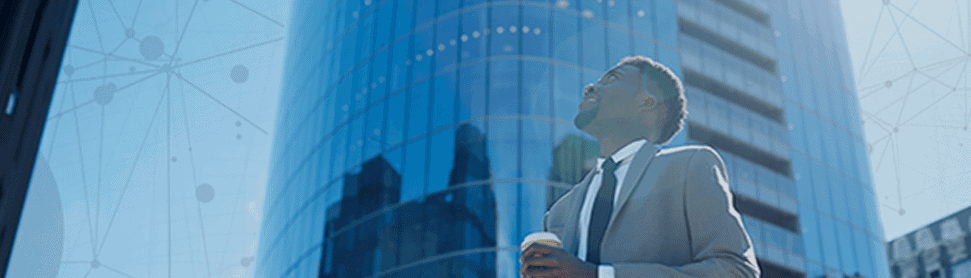
How to Ask a Data-Driven Question
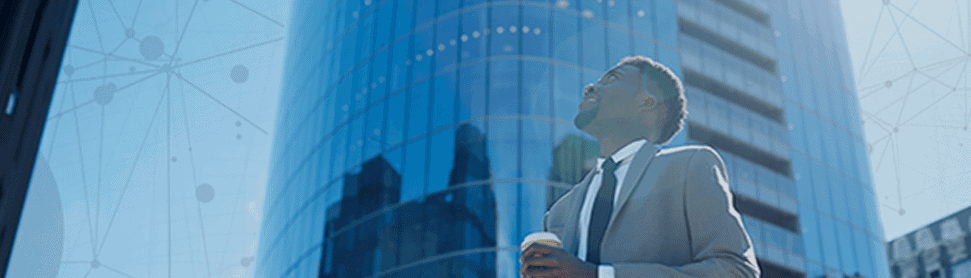
Data has become central to how we run our businesses today. Yet, Gartner reports that as many as 85% of big data projects fail.
According to Harvard Business Review, one reason for this problem is connected to how we receive information: people tend to lend extra authority to numbers appearing on a screen—as opposed to in a journal or nonfiction textbook encountered in a library, for example. After data is filtered via research databases, online, and analyzed via analytics software, we are more likely to trust that information without verifying the data source or appropriateness of the original context. In other words, we should never take data at face value. Instead, we must learn how to ask the right questions.Getting the most out of data means asking a question and allowing the data to inform the answer rather than finding data to support your conclusion.
Why Ask a Data-Driven Question?
Don’t get fooled into trusting insufficient data, for example, by forming an opinion based on one or two criteria turning out how you’d hoped. That’s another way to describe ‘proof by example’—a.k.a. inappropriate generalization: the problematic nature of that type of reasoning is built into the term.
Instead, when a company like Guardian hires data scientists to convert raw data into usable customer insights, they require a repeatable set of tests and results. Implementing such a predictive analytics policy requires testing hypotheses—similar to what is enacted in a scientific lab—hence the term ‘data scientist.’ This type of company policy helps to ‘future-proof’ it, as well, since it increases the chances your business will make better choices in the future based on past results.
When coupled with trusted data, an excellent data-driven question will allow the data to provide the answer. If you don’t start with an appropriate question, the opposite is true— you’ll find a solution you are fishing for, but it shouldn’t be trusted.
So Much Data, So Little Time
Too much data means you didn’t ask the question correctly, and too much data can lead to bad decisions – so don’t go down a rabbit hole of random data.
If there is too much data – you can go down a data rabbit hole and never find the answer you are looking for. This is how companies who have invested in their data get frustrated. At the end of several years, they still can’t trust the answers the data seems to provide.
How do you identify a rabbit hole?
First, we should examine what it’s not: according to Gartner, data literacy is “the ability to read, write, and communicate data in context. It requires understanding data sources and constructs, analytical methods and techniques applied, and the ability to describe the use-case application and resulting value.”
In other words, it is essential to evaluate the validity of data before trusting it, so an excellent analytical process is worth implementing. It involves identifying statistically significant—that is, repeatable and testable—data; data that is verified by multiple trustworthy sources; and data that is found again and again to be accurate and verifiable—rather than a one-hit-wonder of sorts. If it is a one-time phenomenon or only reported by questionable sources, you may have been led down what’s known as a ‘rabbit hole.’ These are often characterized by wild speculation, tall tales, or scientifically unsound ‘data’ presented as evidence but ultimately amounting to fluff or hearsay. Many conspiracy theories can be classified this way.
Start With the Right Questions
Do you know if you’re asking the right questions? And how do you know which questions to ask?
LoadSpring’s approach to asking a good data question first and allowing the question to dictate the data source will provide you with usable data to answer your business questions. Likewise, LoadSpring’s unique data collection, analysis, and cleansing approach—as demonstrated by LoadSpring INSIGHTS—will save time and endless frustration. Get data to a place that is trustworthy before you analyze it.
Data analysis will always rely on human smarts and common sense. Beginning with the right questions is a great example, as in an approach from “data storytelling” author Brent Dykes:
First, answer this question: “What is the problem?” This is the starting point. Note that different stakeholders need answers to various problems. For example, field teams may need to know how to organize onsite resources, while finance’s challenge could be to optimize returns on cash assets, marketing wants to know where to look for new business, and so on.
Second, ask, “What do we want as the outcome?” This is the ideal, desired endpoint. Stakeholders should state their specific goals. For field teams, the aim might be to finish a project on time, within budget, and to scope, while the finance department may want to achieve 6 percent more EBITDA; and marketing may aim for eight new significant accounts this year.
Third, consider, “Which actions are needed?” These actions should take you from the problem to the outcome. Field teams must execute a robust project task schedule based on the above. At the same time, finance needs to develop a model to show the trade-off between risk and return for different cash investments. Marketing aims to generate and prospect a list of sectors or accounts sorted by revenue potential.
Finally, end by asking, “Which measures shall we take?” Measures let you check progress towards outcomes. For field teams, measures could include how well they meet the schedule, milestones, time and money spent on finance, and regular reporting on investments made and their returns. Whereas marketing teams care more about the number of accounts signed and the dollar value of the deals made.
LoadSpring INSIGHTS is the only analytics system that allows you to start with your business question to identify data sources before your data analysis begins.
- LoadSpring INSIGHTS starts with the question – then selects the application and project data that will inform the decision.
- Before making an informed decision, your project data goes through a transparent filtering and configuration process that gets you to a usable data lake (in 90 days!), so you can analyze good data and trust the results.
Ready to take your insights to the next level? LoadSpring INSIGHTS will get your project control application to a clean data lake in three months. And LoadSpring’s project platform, in conjunction with LoadSpring INSIGHTS, will help get you there.
Contact us to take your insights to the next level, starting today.